Table Of Content

Such an experiment allows the investigator to study the effect of each factor on the response variable, as well as the effects of interactions between factors on the response variable. Factorial designs require the experimenter to manipulate at least two independent variables. Imagine you are trying to figure out which of two light switches turns on a light. The dependent variable is the light (we measure whether it is on or off). The first independent variable is light switch #1, and it has two levels, up or down. The second independent variable is light switch #2, and it also has two levels, up or down.
Selecting Factors: Factor and Intervention Component Compatibility
This might result in subadditive or negative interactions in which interventions produce less benefit, or even produce net decreases in benefit, when they co-occur with another intervention(s). This can pose interpretive challenges as it may be difficult to separate the effects of a component per se from the impact of burden. Factorial designs can pose challenges, but they offer important advantages that can offset such challenges. Of course, there is increased efficiency as investigators can screen more components at a reduced expenditure of resources. For instance, RCTs need not have a true placebo or control condition. Thus, two different active treatments might be contrasted with one another in a two-group design, such as a comparison of two different counseling approaches (e.g., skill training vs. supportive counseling), each paired with the same medication.
1 Setting Up a Factorial Experiment
Don't forget about the “scientist” in your Data Scientist title (Part 2) - Towards Data Science
Don't forget about the “scientist” in your Data Scientist title (Part .
Posted: Thu, 06 Jan 2022 08:00:00 GMT [source]
Half Normal Plots for wt% methanol in biodiesel and number of theoretical stages are shown below. The next step is selecting which terms will be analyzed for the responses. Explore Psychology provides information about psychology and mental health. The material found on this site is for informational purposes only and is not intended to be used for diagnosis or treatment purposes. So, the researchers decided to look at how the amount of sleep and caffeine influence test performance. “It is true that when two manipulations are operating simultaneously, it is impossible to disentangle their effects completely,” explain authors Breckler, Olson, and Wiggins in their book Social Psychology Alive.
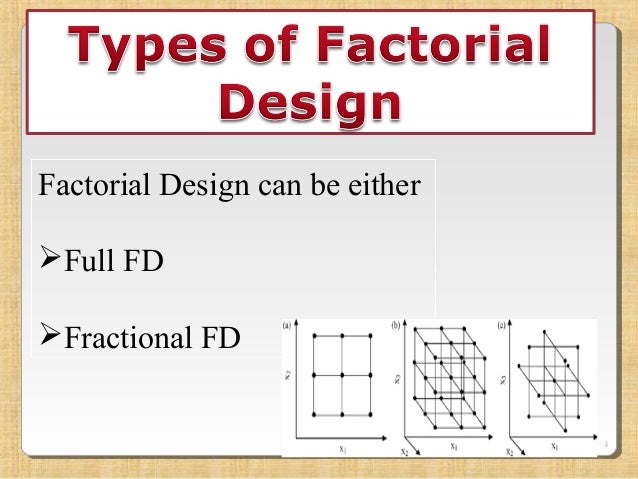
5.2. Assessing Relationships Among Multiple Variables¶
Now we are in a position where we can drop the interactions and reduce this model to a main effects only model. You can see that the C and D interaction plot the lines are almost parallel and therefore do not indicate interaction effects that are significant. However the other two combinations, A and C and A and D, indicate that significant interaction exists. If you just looked at the main effects plot you would likely miss the interactions that are obvious here. We need to think about where the variation occurs within this design.
2.3. Assigning Participants to Conditions¶
Onwards, the minus (−) and plus (+) signs will indicate whether the factor is run at a low or high level, respectively. Frank Yates made significant contributions, particularly in the analysis of designs, by the Yates analysis. Archival data involves using existing records or data, such as medical records, administrative records, or historical documents, as a source of information.
A new multi-factor multi-objective strategy based on a factorial presence-absence design to determine polymer additive ... - ScienceDirect.com
A new multi-factor multi-objective strategy based on a factorial presence-absence design to determine polymer additive ....
Posted: Tue, 18 Oct 2022 02:33:51 GMT [source]
4. Complex Correlational Designs¶
But including multiple independent variables also allows the researcher to answer questions about whether the effect of one independent variable depends on the level of another. This is referred to as an interaction between the independent variables. Schnall and her colleagues, for example, observed an interaction between disgust and private body consciousness because the effect of disgust depended on whether participants were high or low in private body consciousness. As we will see, interactions are often among the most interesting results in psychological research.
Given our assumption that these high-order effects are unlikely to be important, we have little worry that they will contaminate our estimate of the main effects and two-factor interactions. The interaction effects situation is the last outcome that can be detected using factorial design. From the example above, suppose you find that 20 year olds will suffer from seizures 10% of the time when given a 5 mg CureAll pill, while 20 year olds will suffer 25% of the time when given a 10 mg CureAll pill.
From this table, we can see that there is positive correlation for factors A and C, meaning that more sleep and more studying leads to a better test grade in the class. Factor B, however, has a negative effect, which means that spending time with your significant other leads to a worse test score. The lesson here, therefore, is to spend more time sleeping and studying, and less time with your boyfriend or girlfriend. However, the Normal Plot displays whether the effect of the factor is positive or negative on the response. When the difference in response between the levels of one factor is not the same at all the levels of the other factor, there is an interaction between the factors. Regardless of whether the design is between subjects, within subjects, or mixed, the actual assignment of participants to conditions or orders of conditions is typically done randomly.
Interaction Effects
This is where we are headed, a steady progression to designs with more and more factors, but fewer observations and less direct replication. Let's look at another example in order to reinforce your understanding of the notation for these types of designs. Below is a figure of the factors and levels as well as the table representing this experimental space. It should be quite clear that factorial design can be easily integrated into a chemical engineering application. Many chemical engineers face problems at their jobs when dealing with how to determine the effects of various factors on their outputs. For example, suppose that you have a reactor and want to study the effect of temperature, concentration and pressure on multiple outputs.
Thus, to the extent that two ICs affect coping execution or withdrawal severity, their co-occurrence in the experiment could reduce estimates of their main effects via negative interaction. One might think of this as interventions “competing” for a limit subset of participants who are actually capable of change or improvement; in a sense this subsample would be spread across multiple active intervention components. Experimental design provides a structured approach to designing and conducting experiments, ensuring that the results are reliable and valid. In a factorial design, participants are randomly assigned to one of several groups, each of which receives a different combination of two or more independent variables. Interactions occur when the effect of an independent variable depends on the levels of the other independent variable.
The first independent variable will be time since last meal (1 hour vs. 5 hours), and the second independent variable will be how tired someone is (not tired vs very tired). Main effects occur when the levels of an independent variable cause change in the measurement or dependent variable. There is one possible main effect for each independent variable in the design.
A factorial design is a type of experiment that involves manipulating two or more variables. While simple psychology experiments look at how one independent variable affects one dependent variable, researchers often want to know more about the effects of multiple independent variables. For the vast majority of factorial experiments, each factor has only two levels. For example, with two factors each taking two levels, a factorial experiment would have four treatment combinations in total, and is usually called a 2×2 factorial design. In such a design, the interaction between the variables is often the most important.
Assuming that we are designing an experiment with two factors, a 2 x 2 would mean two levels for each, whereas a 2 x 4 would mean two subdivisions for one factor and four for the other. It is possible to test more than two factors, but this becomes unwieldy very quickly. The primary way of doing this is through the statistical control of potential third variables. Instead of controlling these variables by random assignment or by holding them constant as in an experiment, the researcher measures them and includes them in the statistical analysis. Consider some research by Paul Piff and his colleagues, who hypothesized that being lower in socioeconomic status (SES) causes people to be more generous [PKCote+10]. They measured their participants’ SES and had them play the “dictator game.” They told participants that each would be paired with another participant in a different room.
No comments:
Post a Comment